Blog
Explore our insightful articles packed with industry trends, expert advice, and practical solutions to elevate your knowledge.
Sort by:
Showing 12 of 325
- Agentic AI, Agentic AI in Marketing
- Agentic AI, Agentic AI in Marketing
- Agentic AI, Agentic AI in Marketing
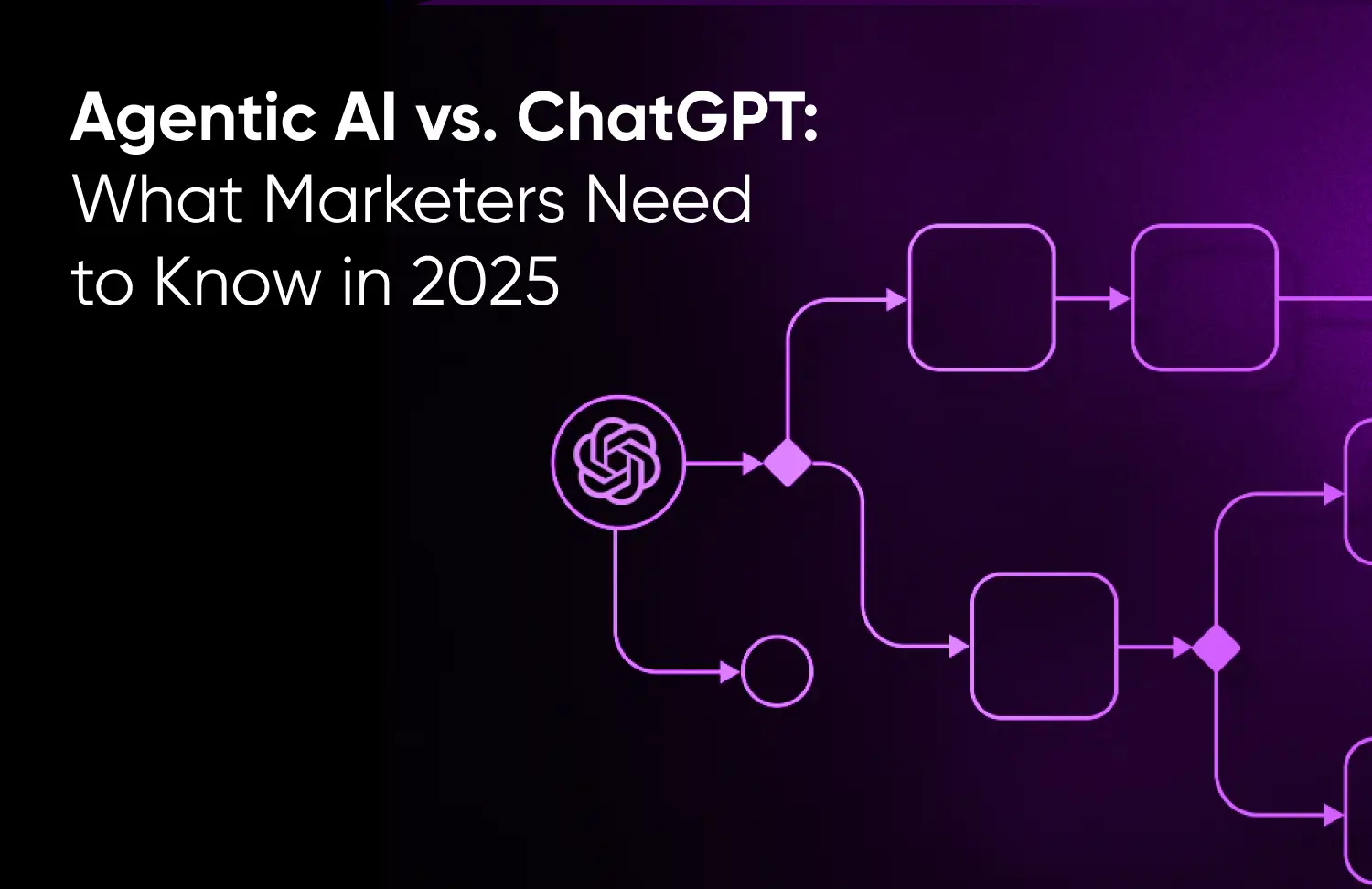
- Agentic AI, Agentic AI in Marketing, Gen AI, Generative AI
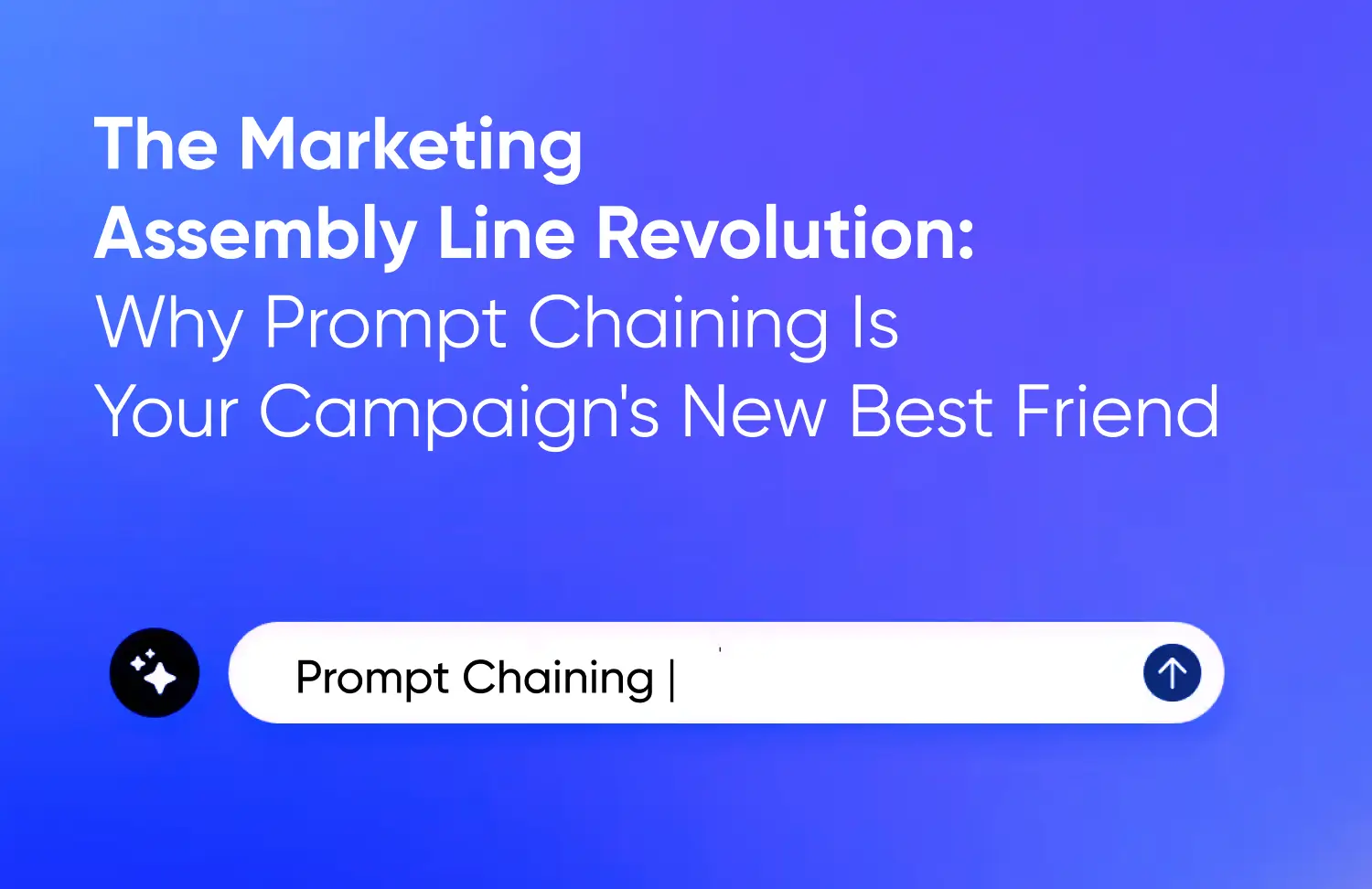
- Agentic AI, Agentic AI in Marketing
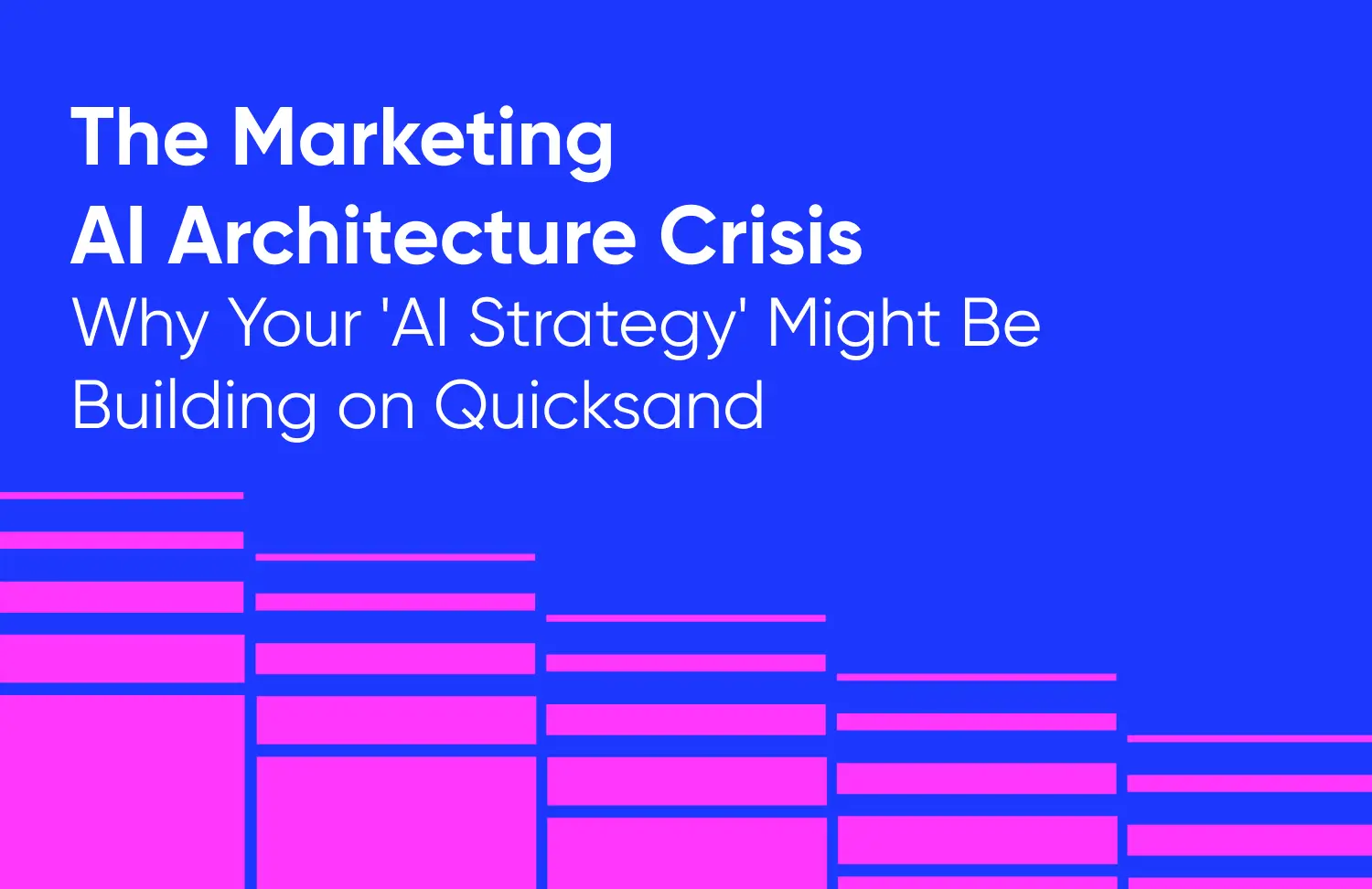
- Agentic AI, Agentic AI in Marketing
- Agentic AI, Agentic AI in Marketing
- Agentic AI, Agentic AI in Marketing
- Agentic AI, Agentic AI in Marketing
- Data Science, Google Analytics 4 (GA4), Google Marketing Platform, Predictive Analytics
- Agentic AI, Agentic AI in Marketing
- Conversion Rate Optimization (CRO)